3 Ways Injection Molders Use Design of Experiments to Improve Processes
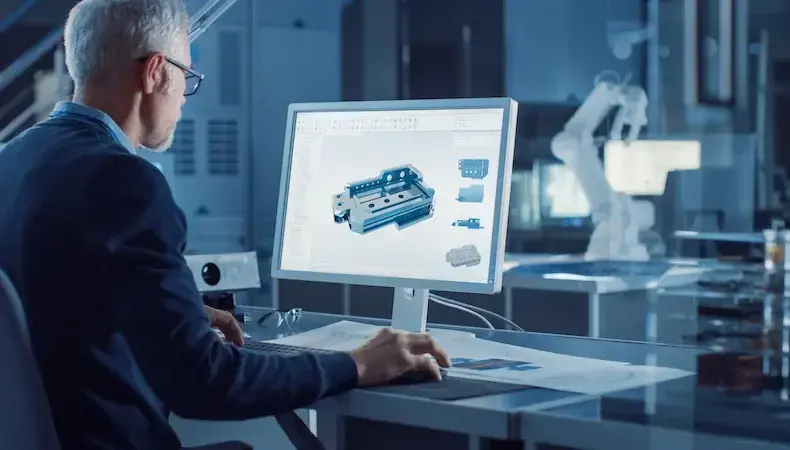
Custom injection molding is, by nature, highly precise. Part performance and reliability hinge on production processes that ensure accurate, consistent outcomes. Being able to deliver on these nuances is often what sets custom injection molders apart — and generally typifies molders with engineers trained in scientific molding.
Within scientific molding lies Design of Experiments (DOE), a branch of applied statistics that uses input and output variables in controlled tests to determine and analyze certain values, measurements, and external influences. The resulting data serves as the basis for mathematical modeling that gives engineers insight into potential issues — and their resolutions, be it recommended changes or further required testing.
The ultimate goal of DOE is identifying and maintaining the ideal injection molding process window by maximizing performance, efficiency, and reliability improvements. The following design of experiments examples help illustrate how Kaysun leverages DOE, and how DOE impacts these three key areas.
Design of Experiments Examples
1. Improved Performance
Generally speaking, injection molding process performance is defined by variables (inputs) and the effect of those variables (outputs). DOE is fundamental in identifying the relation between inputs and outputs, and which influence the injection molding process the most.
Pack pressure, mold temperature, and cooling time are among the most popular variables analyzed, but DOE doesn’t limit engineers to working with one variable at a time. Analyzing multiple variables concurrently allows for upfront discovery of critical interactions that could cause potential dimensional or cosmetic variation in production. Further, the statistical approach helps engineers hone in on process stabilization that leads to highly repeatable parts.
2. Improved Efficiency
DOE allows engineers to manipulate variables and understand the resulting dimensional output in real-time, outside of the molding press.
Process variables can be adjusted using a statistical software program. As such, excesses in time, materials, and rework are virtually eliminated, streamlining the project development phase. In turn, process optimization is realized which translates to faster time to market and competitive advantages for OEMs.
3. Improved Reliability
In-depth data gathered from analyzing variables against molding processes provides a better understanding of which combination delivers optimal repeatability. Engineers then have the latitude to focus on and control that particular variable grouping in order to verify a highly reliable and repeatable injection molding process.
For instance, let’s say an engineer concludes that pack pressure has the greatest effect on a critical controlled dimension. With DOE, cavity pressure transducers are used to closely monitor pressure stability over the course of a run.
Or, should the monitoring prove pack pressure isn’t an influential variable, DOE allows for a seamless pivot to other variable monitoring to determine possible outliers. DOE helps engineers concentrate on variable monitoring instead of guesswork to ultimately develop a reliable and repeatable process.
When a project calls for tolerances as tight as +/- .001 inches — as many custom injection-molding applications do — there is no room for error.
As illustrated by the design of experiments examples, scientific molding and its related processes offer engineers precise production optimization solutions that accommodate even the most demanding part performance, efficiency, and reliability needs.
Learn more in our white paper, An OEM’s Guide to Scientific Molding. Click the button below to download your copy now.
Subscribe
TO OUR BLOG
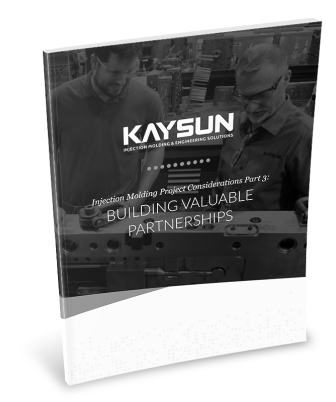
How Do Injection Molder Partnerships Influence Project Costs?
You Might Also Like...
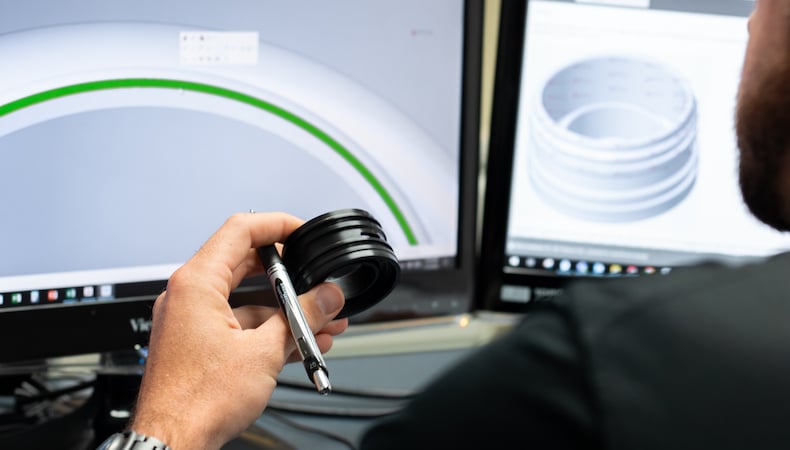
How RJG Master Molder I/II Defines Injection Molding Excellence
In custom injection molding, quality and precision are both an expectation and a g…
READ MORE
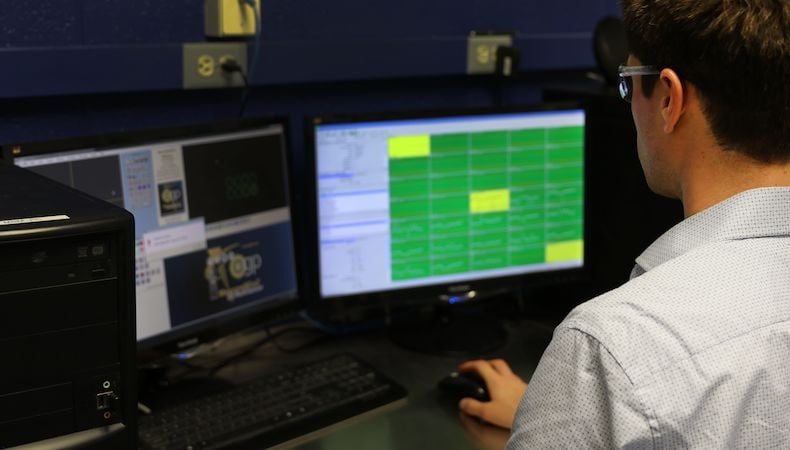
How Today’s Manufacturers Benefit from Scientific Injection Molding
As technologies and demand rapidly increase the competition in many industries, th…
READ MORE
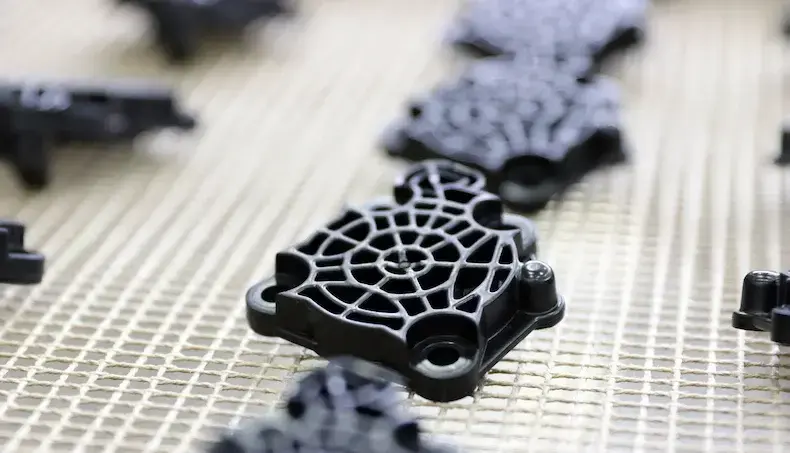
3 Engineering Support Services Your Injection Molder Must Offer
There’s no denying that pandemic-related, weather-influenced, and manmade disrupti…
READ MORE